The ACL (Annual Meeting of the Association for Computational Linguistics) is one of the most authoritative international conferences in the field of Natural Language Processing (NLP) within the realm of artificial intelligence, and it is classified as a CCF A-level conference. The first author of the paper accepted by ACL2024 is Li Jiang, a doctoral student supervised by Prof. Gao Guanglai. This paper focuses on the issue of knowledge graph completion across multiple domains for the first time. This research work has received funding support from the National Natural Science Foundation of China and the autonomous region's key research and development and achievement transformation plan projects. The National-Regional Joint Engineering Research Center for Intelligent Information Processing Technology in Mongolian Language has provided software and hardware support for this research.
"Learning Low-dimensional Multi-domain Knowledge Graph Embedding via Dual Archimedean Spirals"
Authors: Li Jiang, Su Xiangdong, Zhang Fujun, Gao Guanglai
Affiliation: Inner Mongolia University
Knowledge graph embedding, which represents entities and relationships as low-dimensional vectors, has been widely used in link prediction tasks. In practical applications, knowledge graphs often cover multiple domains, posing significant challenges to the representation. However, existing KGE methods rarely impose explicit domain constraints on the embedding distribution in multi-domain knowledge graphs, leading to overlapping embeddings of different domains and reduced performance in link prediction.
To address this challenge, we propose "Dual Archimedean Spirals Knowledge Graph Embedding" (DuASE), a low-dimensional embedding model specifically designed for multi-domain knowledge graphs. The motivation for DuASE stems from our discovery that relationship types can be used to distinguish entities from different domains. In the context of knowledge graphs, the term "domain" is used to describe the specific subject area or categories of knowledge covered or represented by the graph, referring to the scope of entities, concepts, and relationships included in the graph. The differences between different domains are mainly manifested in the types of entities and relationships. Since most knowledge graphs do not provide entity type information, we mainly rely on relationship types to differentiate domain knowledge and avoid the issues of embedding overlap.
Specifically, DuASE encodes entities with the same relationship along the same Archimedean spiral, effectively distinguishing entities from different domains. To prevent overlap of cross-domain embeddings, DuASE further uses a regularization function to ensure that the head and tail entities within the same triplet spiral along their respective domain spaces. Therefore, when modeling multi-domain knowledge graphs, DuASE can more accurately capture domain information and the dependencies between entities, thus optimizing the embedding representation of the knowledge graph. Additionally, we have constructed a multi-domain dataset n-MDKG and verified the effectiveness of DuASE on n-MDKG and three other benchmark datasets.
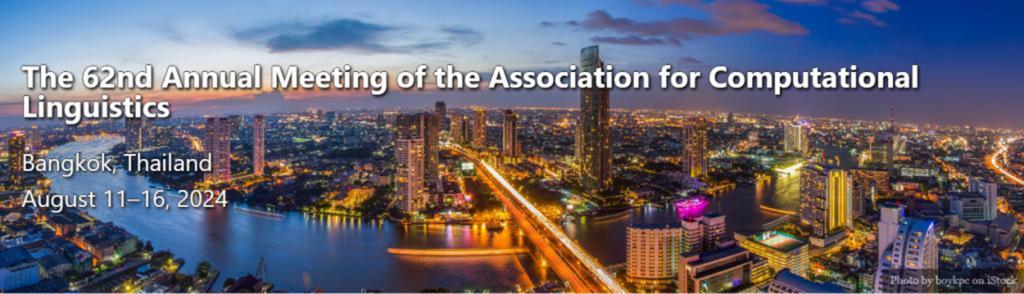